
Learn more about how we’re making progress towards our sustainability commitments through the Sustainable by design blog series, starting with Sustainable by design: Advancing the sustainability of AI.
Earlier this summer, my colleague Noelle Walsh published a blog detailing how we’re working to conserve water in our datacenter operations: Sustainable by design: Transforming datacenter water efficiency, as part of our commitment to our sustainability goals of becoming carbon negative, water positive, zero waste, and protecting biodiversity.
At Microsoft, we design, build, and operate cloud computing infrastructure spanning the whole stack, from datacenters to servers to custom silicon. This creates unique opportunities for orchestrating how the elements work together to enhance both performance and efficiency. We consider the work to optimize power and energy efficiency a critical path to meeting our pledge to be carbon negative by 2030, alongside our work to advance carbon-free electricity and carbon removal.
Explore how we’re advancing the sustainability of AI
Explore our three areas of focus
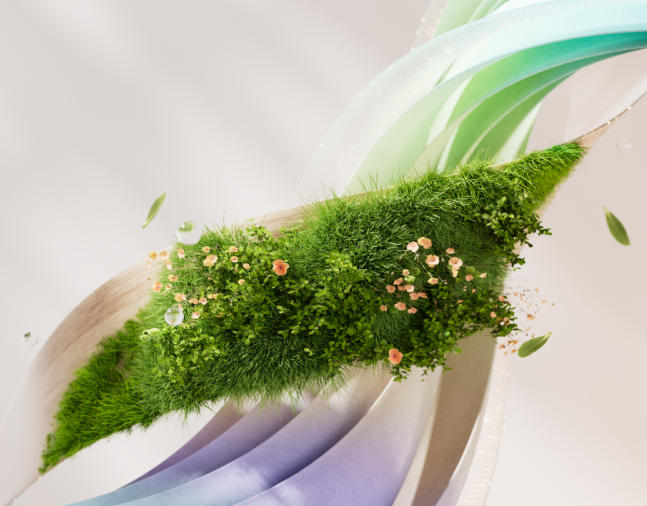
The rapid growth in demand for AI innovation to fuel the next frontiers of discovery has provided us with an opportunity to redesign our infrastructure systems, from datacenters to servers to silicon, with efficiency and sustainability at the forefront. In addition to sourcing carbon-free electricity, we’re innovating at every level of the stack to reduce the energy intensity and power requirements of cloud and AI workloads. Even before the electrons enter our datacenters, our teams are focused on how we can maximize the compute power we can generate from each kilowatt-hour (kWh) of electric power.
In this blog, I’d like to share some examples of how we’re advancing the power and energy efficiency of AI. This includes a whole-systems approach to efficiency and applying AI, specifically machine learning, to the management of cloud and AI workloads.
Driving efficiency from datacenters to servers to silicon
Maximizing hardware utilization through smart workload management
True to our roots as a software company, one of the ways we drive power efficiency within our datacenters is through software that enables workload scheduling in real time, so we can maximize the utilization of existing hardware to meet cloud service demand. For example, we might see greater demand when people are starting their workday in one part of the world, and lower demand across the globe where others are winding down for the evening. In many cases, we can align availability for internal resource needs, such as running AI training workloads during off-peak hours, using existing hardware that would otherwise be idle during that timeframe. This also helps us improve power utilization.
We use the power of software to drive energy efficiency at every level of the infrastructure stack, from datacenters to servers to silicon.
Historically across the industry, executing AI and cloud computing workloads has relied on assigning central processing units (CPUs), graphics processing units (GPUs), and processing power to each team or workload, delivering a CPU and GPU utilization rate of around 50% to 60%. This leaves some CPUs and GPUs with underutilized capacity, potential capacity that could ideally be harnessed for other workloads. To address the utilization challenge and improve workload management, we’ve transitioned Microsoft’s AI training workloads into a single pool managed by a machine learning technology called Project Forge.
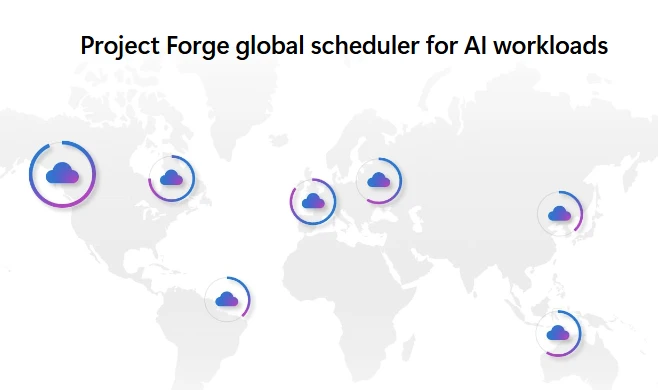
Currently in production across Microsoft services, this software uses AI to virtually schedule training and inferencing workloads, along with transparent checkpointing that saves a snapshot of an application or model’s current state so it can be paused and restarted at any time. Whether running on partner silicon or Microsoft’s custom silicon such as Maia 100, Project Forge has consistently increased our efficiency across Azure to 80 to 90% utilization at scale.
Safely harvesting unused power across our datacenter fleet
Another way we improve power efficiency involves placing workloads intelligently across a datacenter to safely harvest any unused power. Power harvesting refers to practices that enable us to maximize the use of our available power. For example, if a workload is not consuming the full amount of power allocated to it, that excess power can be borrowed by or even reassigned to other workloads. Since 2019, this work has recovered approximately 800 megawatts (MW) of electricity from existing datacenters, enough to power approximately 2.8 million miles driven by an electric car.1
Over the past year, even as customer AI workloads have increased, our rate of improvement in power savings has doubled. We’re continuing to implement these best practices across our datacenter fleet in order to recover and re-allocate unused power without impacting performance or reliability.
Driving IT hardware efficiency through liquid cooling
In addition to power management of workloads, we’re focused on reducing the energy and water requirements of cooling the chips and the servers that house these chips. With the powerful processing of modern AI workloads comes increased heat generation, and using liquid-cooled servers significantly reduces the electricity required for thermal management versus air-cooled servers. The transition to liquid cooling also enables us to get more performance out of our silicon, as the chips run more efficiently within an optimal temperature range.
A significant engineering challenge we faced in rolling out these solutions was how to retrofit existing datacenters designed for air-cooled servers to accommodate the latest advancements in liquid cooling. With custom solutions such as the “sidekick,” a component that sits adjacent to a rack of servers and circulates fluid like a car radiator, we’re bringing liquid cooling solutions into existing datacenters, reducing the energy required for cooling while increasing rack density. This in turn increases the compute power we can generate from each square foot within our datacenters.
Learn more and explore resources for cloud and AI efficiency
Stay tuned to learn more on this topic, including how we’re working to bring promising efficiency research out of the lab and into commercial operations. You can also read more on how we’re advancing sustainability through our Sustainable by design blog series, starting with Sustainable by design: Advancing the sustainability of AI and Sustainable by design: Transforming datacenter water efficiency.
For architects, lead developers, and IT decision makers who want to learn more about cloud and AI efficiency, we recommend exploring the sustainability guidance in the Azure Well-Architected Framework. This documentation set aligns to the design principles of the Green Software Foundation and is designed to help customers plan for and meet evolving sustainability requirements and regulations around the development, deployment, and operations of IT capabilities.
1Equivalency assumptions based on estimates that an electric car can travel on average about 3.5 miles per kilowatt hour (kWh) x 1 hour x 800.