
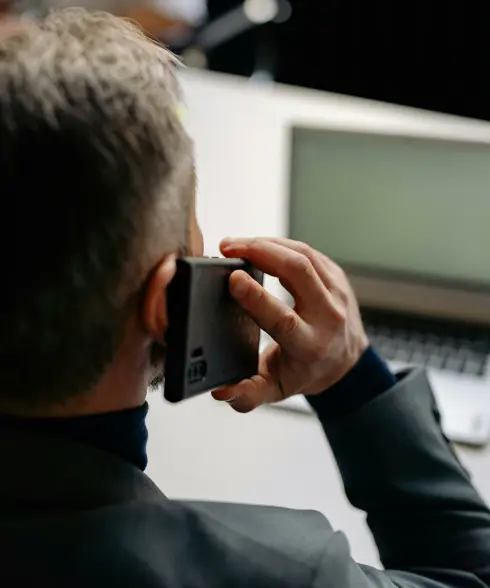
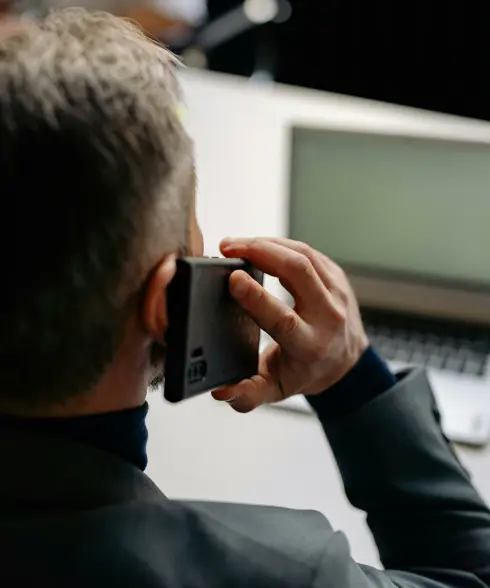
The artificial intelligence (AI) landscape is experiencing a transformative moment in voice technology. Anthropic’s recent release demonstrates breakthrough capabilities in natural conversation, with an enhanced ability to understand context and nuanced speech patterns. Meanwhile, OpenAI’s latest developments focus on more human-like voice synthesis and improved recognition across diverse accents and speaking styles. These advancements signal a shift from basic command-and-response systems to more sophisticated, context-aware voice interactions.
As voice AI technology advances, integration into everyday applications has accelerated. The applications span countless industries, from healthcare systems requiring precise medical terminology recognition to financial services needing secure voice authentication. However, this rapid adoption brings new challenges for development teams, ensuring these systems work reliably and inclusively for all users.
The complexity of human speech and the increasing sophistication of AI voice technology demands a structured approach to testing and validation. While AI models have become more capable of understanding natural language, they must still be thoroughly tested across various scenarios and user populations. The following four critical considerations emerge as essential focal points for development teams working to integrate voice capabilities into their applications.
-
Language Diversity and Evolution
Voice recognition systems must adapt to the constantly evolving nature of human speech. Language variations extend far beyond simple accent differences. Modern systems must account for generational language patterns and emerging slang while managing regional dialects and cultural expressions simultaneously. The challenge of brand name pronunciation adds another layer of complexity, as these often vary significantly across different populations. Social and demographic factors further influence speech patterns, creating a multifaceted landscape that voice recognition systems must navigate. With over 4.2 billion digital voice assistants in use worldwide, accurate language processing has become crucial for user adoption. Industry research indicates that 30% of users report dissatisfaction with current voice assistants, primarily due to recognition accuracy issues.
-
Contextual Understanding
Modern voice applications must move beyond simple command recognition to understand user intent within context. Development teams must build systems capable of situational awareness in voice processing, handling multiple interpretations of similar phrases, and recognizing underlying user intent. When misunderstandings occur, these systems should continuously learn from user interactions and implement context-based error correction. Testing must verify that systems can maintain accuracy across various scenarios and user behaviors, requiring comprehensive testing with diverse user groups rather than controlled laboratory conditions.
-
Accessibility and Inclusion
Voice technology must serve all users, regardless of speech patterns or conditions. Testing protocols must address the full spectrum of speech variations, including medical conditions that affect speech, age-related voice characteristics and non-standard pronunciation patterns. Systems must handle varying speech velocities and interrupted speech patterns without losing accuracy or functionality. Real-world testing conditions help identify these challenges, ensuring voice applications work for everyone daily. This is especially important given how much of a positive impact voice technology products can have for the disability community, as it has the potential to eliminate a lot of the friction many tend to experience with more traditional interaction tools.
-
Implementation Strategy
Development teams should establish diverse testing groups representing different demographics and speech patterns to integrate these considerations successfully. Continuous feedback loops from actual users provide vital data for improvement, while regular model updates ensure systems stay current with evolving language patterns. Rigorous testing in various environmental conditions and careful verification of accessibility compliance complete the testing framework.
The Next Frontier Will Include AI EQ
As AI voice technology evolves, testing methodologies must adapt. The focus should shift from simple command recognition to understanding complex user interactions and context. Success requires thorough testing with diverse user groups and continuous refinement based on real-world usage patterns.
The next frontier in voice AI testing will likely involve emotional intelligence and conversational nuance. As systems become more sophisticated at detecting vocal patterns and emotional states, testing protocols must expand to verify appropriate responses to user sentiment and conversational context.
Teams integrating voice AI must prioritize inclusive design principles and comprehensive testing strategies. This approach ensures voice technology effectively serves all users, regardless of how they speak or interact with these systems. With proper testing and validation, voice AI can fulfill its promise of making technology more accessible and intuitive for everyone.